Introduction
According to the World Bank, Kenya is classified as a lower-middle-income economy with a gross national income of $1,460 ( USD). As such, it is ideally situated to leverage the work of nonprofits to bring about positive economic growth. Unfortunately, nonprofits often lack the financial and personnel resources to determine analytically where those resources are best allocated. This article aims to alleviate that problem for nonprofits in Kenya by providing a general framework of the binding constraints affecting Kenyan businesses. For example, a binding constraint in the manufacturing sector could be a lack of electricity infrastructure. Even if there is enough capital to produce at a certain level, this electricity problem could hold a business back from expanding. It is my hope that this article and its contents can be used as a resource to assist both nonprofits and businesses in Kenya as they attempt to grow. Additionally, the results of this article may be of use to nonprofits aimed at funding startups as it provides a general guide to focus their efforts.
The article follows the following structure: a brief overview of the data used, general descriptive statistics including graphical representations, and an analysis of the findings
Data
This study uses World Bank Enterprise Survey data on 781 domestic Kenyan businesses. Firms are separated into three categories: small, medium, and large. Small firms are comprised of 5-19 employees. Medium firms are comprised of 20-99 workers. Large firms have 100+ employees. The World Bank Enterprise Survey data excludes firms with less than 5 employees along with the informal sector. It is intended to be representative of the private sector economy excluding agriculture.
The data includes ranking for 16 different obstacles on an ordinal scale. We use the framework proposed by Hausmann, Rodrik, and Velasco to identify binding constraints and classify the obstacles accordingly. Figure 1 details a flowchart summarizing the HRV framework.
Figure 1:
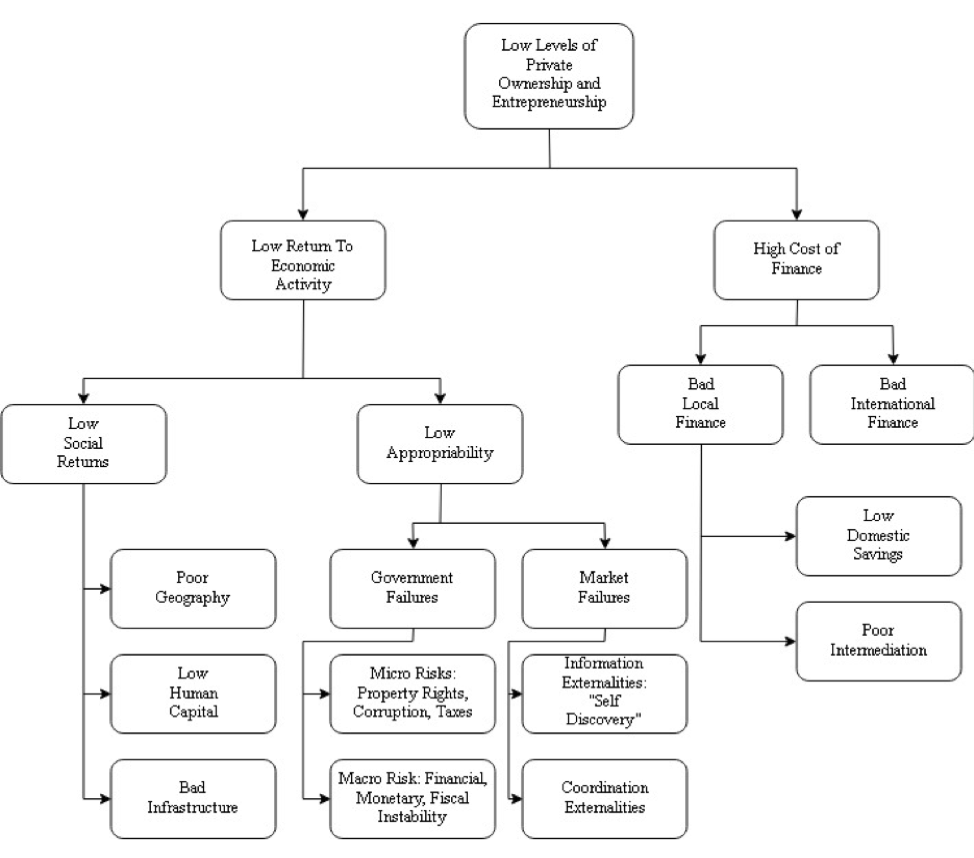
Descriptive Statistics
Figure 2 shows the proportion of Kenyan firms that identify issues as either major or very severe obstacles. From the figure it is clear that “Practices of Competitors in the Informal Sector”, “Inadequately Educated Workforce”, “Courts”, and “Access to Land” comprise the most significant obstacles for firms in Kenya. “Tax Administration”, “Tax Rates”, “Business Licensing and Permits”, and “Access to Finance” are not as significant. In particular, the “Access to Financing” result is intuitively surprising due to the commonality of poor financial
Figure 2:
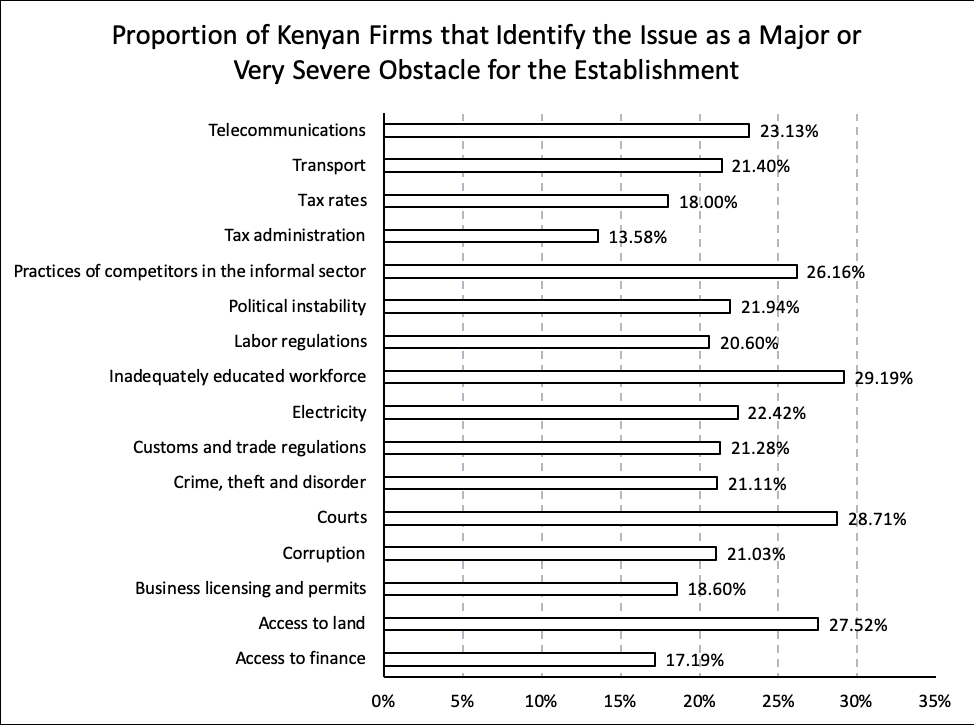
Our dataset is comprised of three different sizes of firms and it would be naïve to assume that they all face identical constraints. What constitutes a binding constraint for a small firm might not be an issue for a large firm. Table 1 shows the breakdown of the top five most identified obstacles for firms in Kenya according to size. From this table it is clear that Practices of Competition in the Informal Sector, Electricity, and Tax Rates are similar obstacles for all three firm sizes while Corruption, Access to Finance, Political Instability, and Customs and Trade Regulations have different impacts on firms according to their size. The fact that only three obstacles are common across all three firm sizes suggests that analyzing the binding constraints of a firm while taking into account its size is probably the most prudent method. Note that access to finance only appears among the biggest problems for small firms. This could be a problem area where nonprofits could help by focusing on microloans in Kenya. It demonstrates an unmet demand from smaller firms for capital. One firm that is already focusing their efforts on microloans is Kiva Zip[1]. Started in 2005, Kiva Zip provides loans as small as $50 dollars to businesses in Kenya’s capital city Nairobi. These loans are devoid of both interest and fees with 100% of the process being able to be conducted via a simple mobile phone. Initial results of Kiva Zip have been very promising with a 90% repayment rate and a tripling of average monthly income among recipients (UNHCR 2018). Additionally, this fact can inform our choice of model since its appearance in only one list suggests it may be a binding constraint keeping small businesses from growing in size.
Table 1:
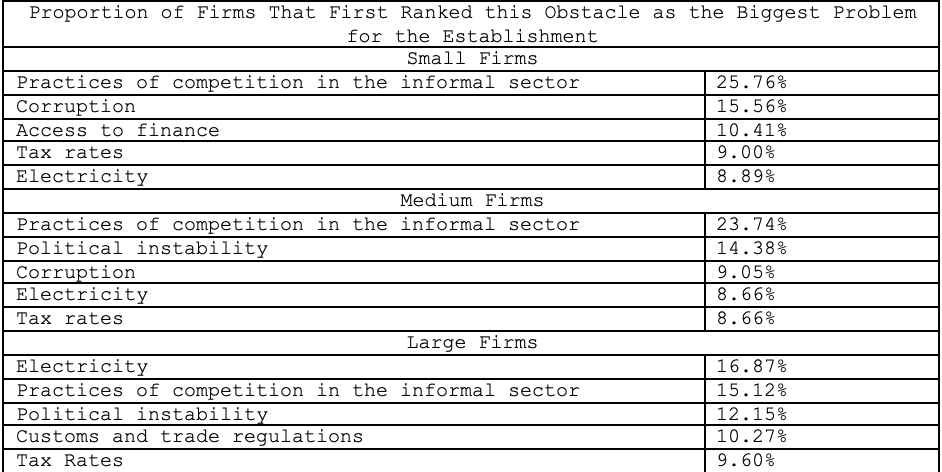
Next, we break down each obstacle by firm size and its ranking (ordinal scale of no obstacle, minor obstacle, moderate obstacle, major obstacle, and very severe obstacle). Figure 3 demonstrates the different rankings of obstacles for four of the sixteen categories. Based on the figure, it is clear that different sized firms face varying levels of obstacles. Figures 4-6 follow the same setup and a similar conclusion can be drawn.
Figure 3:
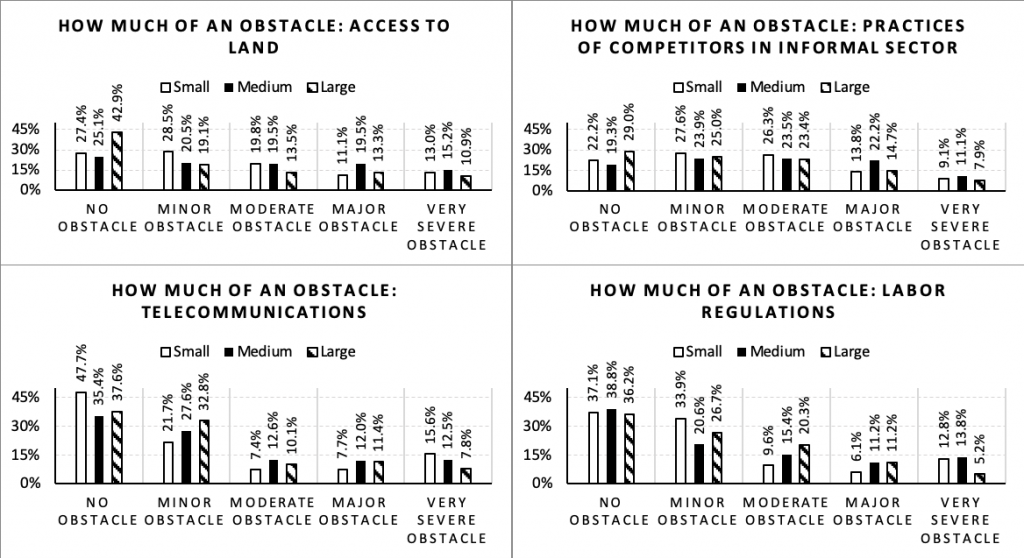
Figure 4:
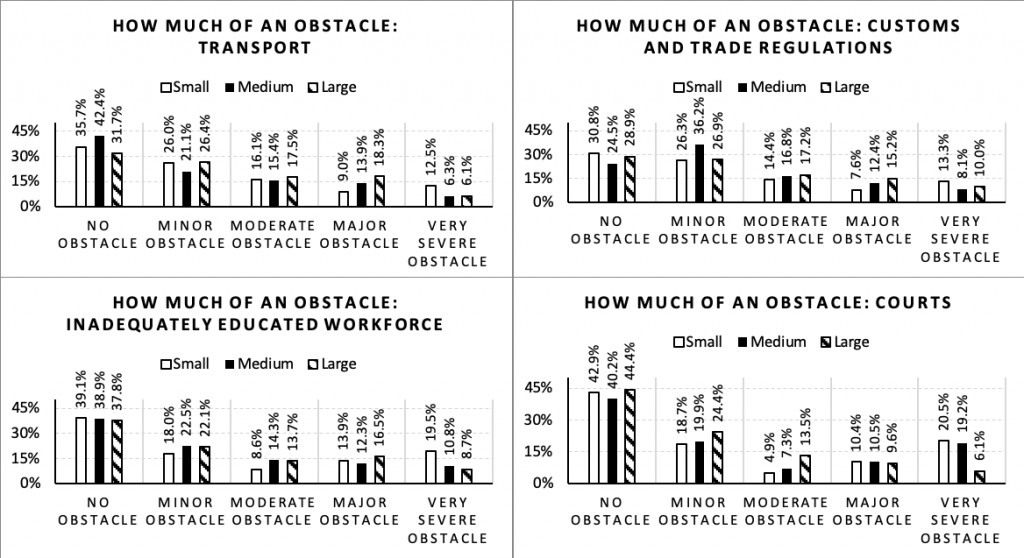
Figure 5:
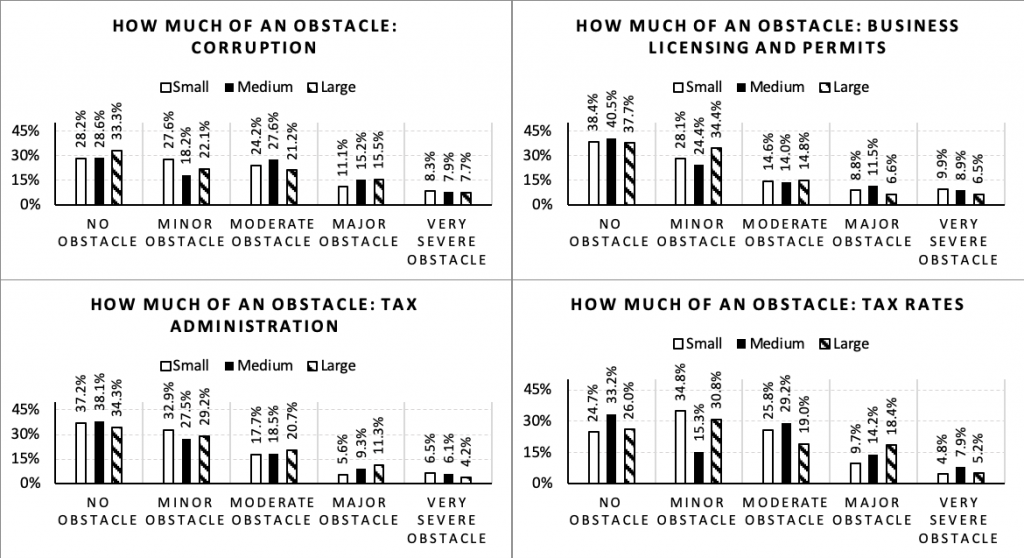
Figure 6:
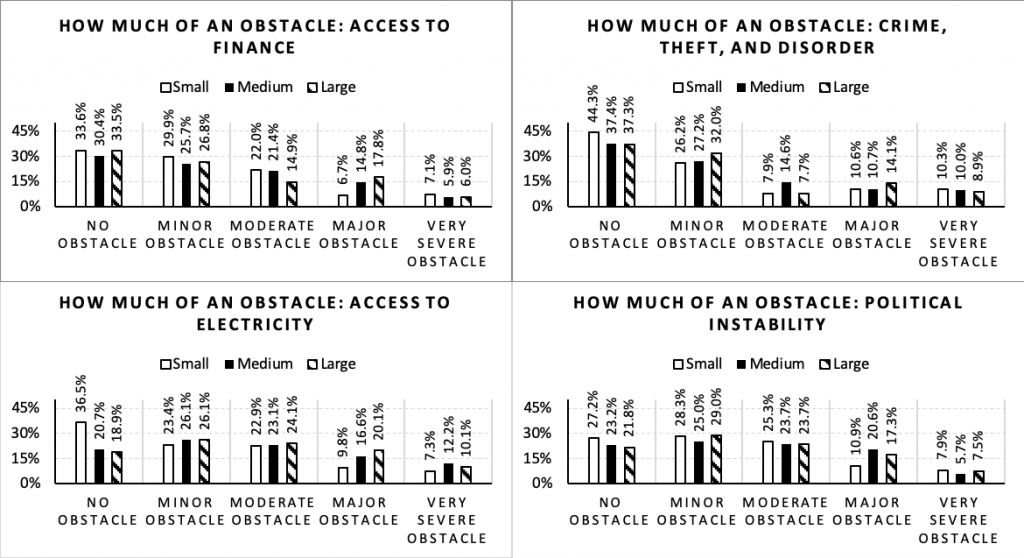
From these figures a general idea of which constraints are likely to be binding in Kenya for various firm sizes can be identified. Upon applying our model, we will be able to get a more specific idea of the extent to which each obstacle constitutes a constraint but the initial descriptive statistics give us a general idea. For small firms, we expect to see political instability, tax rates, corruption, practices of competitors in the informal sector, and access to land to be binding. For medium firms, we observe political instability, access to electricity, corruption, customs and trade regulations, and practices of competitors in the informal sector. Large firms consistently rank political instability, access to electricity, tax rates, customs and trade regulations, and practices of competitors in the informal sector as obstacles. This also gives us an idea of which obstacles are unlikely to be binding constraints for firms. For example, courts are rarely ranked as an obstacle and are unlikely to be an area determining growth for Kenyan firms.
From these figures a general idea of which constraints are likely to be binding in Kenya for various firm sizes can be identified. Upon applying our model, we will be able to get a more specific idea of the extent to which each obstacle constitutes a constraint. Before moving to that step, we can get a general idea just from our descriptive analysis.
For small firms, we expect to see political instability, tax rates, corruption, practices of competitors in the informal sector, and access to land to be binding. For medium firms, we observe political instability, access to electricity, corruption, customs and trade regulations, and practices of competitors in the informal sector. Large firms consistently rank political instability, access to electricity, tax rates, customs and trade regulations, and practices of competitors in the informal sector as obstacles. This also gives us an idea of which obstacles are unlikely to be binding constraints for firms. For example, courts are rarely ranked as an obstacle and are unlikely to be an area standing in the way of growth for Kenyan firms.
Conclusion
Economic growth is never a certainty, and equitable growth across firms of all sizes is even less certain. This article provides insight into the binding constraints in Kenya with the intention of assisting nonprofits operating there. In future posts, an ordered probit model will be applied to the Enterprise Survey data to more rigorously o estimate the constraints. Additionally, a technical article detailing the use of STATA in this series will also be published. Particular focus will be given to the use of the cmp command and the evaluation of valid instrumental variables in the ordered probit model. All data is courtesy of World Bank Enterprise Survey (http://www.enterprisesurveys.org/data/exploreeconomies/2013/kenya).
[1] For more information regarding Kiva Zip and their impact on the Kenyan Economy see https://blog.kiva.org/blog/kiva-zip-kenya-reaches-a-new-milestone-1000-life-changing-loans and https://www.unhcr.org/54edf4479.pdf
Comments